Jeremy Entner, Ph.D., joined Cornell’s Department of Statistics and Data Science as a Lecturer in 2019, where he teaches several courses including “Biological Statistics,” “The Theory of Interest,” and “Statistics for Risk Modeling.” He previously spent six years at the University of Tennessee at Martin teaching courses on mathematics and statistics. Dr. Entner holds a B.S. and M.A. in Mathematics from SUNY Brockport. He earned his Ph.D. in Mathematics with an Emphasis on Statistics from Syracuse University.
Data Science EssentialsCornell Certificate Program
Overview and Courses
In recent years, the field of data science has taken off, as every industry and function increasingly relies on data-driven insights to make decisions.
The statistical programming language R is widely used in data science and understanding the fundamentals of how it works can be helpful, whether you’re considering a career in data science or looking to better communicate with data scientists on your team. In this certificate program, you will develop an essential foundation in R programming skills, then use those skills to understand and summarize data.
In the first course, you will study R programming principles and use R for data manipulation, visualization, and sampling. Building on your skills, you will summarize and visualize real data sets, draw conclusions from those data, and evaluate the uncertainty surrounding those conclusions. Throughout the process, you will develop hypotheses about your data, then use simulations and statistical techniques to evaluate your hypotheses. You will also practice using the Tidyverse open-source R packages to clean and organize your data sets. Finally, you will have the opportunity to manipulate and visualize data using more advanced techniques.
This certificate will ultimately introduce you to the fundamentals of data science and enhance your ability to draw meaningful conclusions from data.
System requirements: This course contains a virtual programming environment that is compatible with Chrome, Firefox, or Internet Explorer.
The courses in this certificate program are required to be completed in the order that they appear.Course list
When you think about what data analysts and data scientists do on a day-to-day basis, you might have a general understanding of types of conclusions they make, but how do they arrive at those conclusions? The statistical programming language R is widely used in data science; understanding the basics of how it works can help you manipulate and visualize data in a quick, flexible manner, and it may improve your communication with data scientists on your team.
In this course, you will explore the basics of statistical programming and develop R skills. As you hone your ability to use commands in R, you will combine those basic skills to complete more complex tasks, such as data manipulation and visualization. Finally, you will examine how to repeat tasks in R, which makes it easier to manipulate large data sets. This course involves many hands-on coding exercises to help you gain confidence in your newfound programming skills.
System requirements: This course contains a virtual programming environment that does not support the use of Safari, Edge, tablets, or mobile devices. Please use Chrome, Firefox, or Internet Explorer on a computer for this course.
- Jan 22, 2025
- Mar 5, 2025
- Apr 16, 2025
- May 28, 2025
- Jul 23, 2025
- Sep 17, 2025
- Nov 12, 2025
The real world is extremely complex, and revealing the patterns that underlie these complexities can be challenging. However, unlocking the power of a data set can provide you with remarkable insights and help guide decision-making. This course will prepare you to use summarization and visualization techniques to reveal patterns in real-world data, using examples from a variety of disciplines, including business and medicine.
In this course, Professor Basu will guide you as you begin to understand key data collection principles and how to make conclusions from data. Choosing which analyses to use depends on your question, so you will use a framework to help you choose which methods to use with your data. Then, you will use R to perform exploratory data analyses, which will allow you to identify key patterns and trends in a ready-to-analyze data set. You will also learn the importance of quantifying the uncertainty associated with your results, and how to measure variability in your data. This course involves many hands-on coding exercises in R to help you gain confidence in your programming skills.
System requirements: This course contains a virtual programming environment that does not support the use of Safari, Edge, tablets, or mobile devices. Please use Chrome, Firefox, or Internet Explorer on a computer for this course.
“Exploring Data Sets With R” must be completed prior to starting this course.
- Dec 25, 2024
- Feb 5, 2025
- Mar 19, 2025
- Apr 30, 2025
- Jun 11, 2025
- Aug 6, 2025
- Oct 1, 2025
- Nov 26, 2025
Data scientists make decisions by inferring the characteristics of a large population based on the characteristics of samples from that population. Basing a decision on samples is necessary since it would not be possible to measure every individual or unit in a population. However, it also means that data scientists need to consider the potential variability among samples before using those samples to make conclusions about the population. The variability across samples leads to uncertainty in decision-making, and understanding and quantifying that uncertainty is a key aspect of data science.
Throughout this course, Professor Basu will guide you through the nuances of understanding and quantifying the uncertainty around your results, and through making decisions in the face of that uncertainty. In data science, simulations offer a powerful framework with which to understand the uncertainty around your data, so you will learn to perform simulations in R and use a simulation-based framework to quantify uncertainty when studying the relationship between categorical variables. You will also use resampling techniques to understand numerical variables and compare their summary statistics across different levels of a categorical variable. Often, data scientists search for relationships between numerical variables and use one numerical variable to predict another numerical variable, and you will do this by building a prediction rule with linear regression while keeping the uncertainty of your results in mind. Finally, you will use the errors from linear regression to compare prediction rules and determine which prediction rules fit your data best. This course involves many hands-on coding exercises in R which will help you gain confidence in your programming skills.
System requirements: This course contains a virtual programming environment that does not support the use of Safari, Edge, tablets, or mobile devices. Please use Chrome, Firefox, or Internet Explorer on a computer for this course.
“Exploring Data Sets With R” and “Summarizing and Visualizing Data” must be completed prior to starting this course.
- Jan 8, 2025
- Feb 19, 2025
- Apr 2, 2025
- May 14, 2025
- Jun 25, 2025
- Aug 20, 2025
- Oct 15, 2025
- Dec 10, 2025
Data scientists use data collected from the real world to answer questions and solve problems that would otherwise be intractable. But since the world is complex, data collected to describe the world can also be complex, which makes it messy and difficult to work with. To successfully analyze data, data scientists need to spend time cleaning — or organizing and manipulating — their data to put it into a form that is easier to work with and understand.
In this course, you will delve into the world of data cleaning by presenting and manipulating your data with the Tidyverse in R. You will organize data by selecting only the variables you're interested in, creating new groups of data, and summarizing data in a way that makes sense for the questions you're trying to ask. You will also create high-quality plots to quickly summarize complex data. You will become familiar with the concept of tidy data and organize data sets in a way that allows for the most efficient analysis. Finally, you will work with data types of more complexity so that you can answer increasingly difficult questions as you take your new skills into your workplace. You will practice all these skills by working with four real-world, complex data sets. This course involves many hands-on coding exercises that will help you take your programming skills to the next level.
System requirements: This course contains a virtual programming environment that does not support the use of Safari, Edge, tablets, or mobile devices. Please use Chrome, Firefox, or Internet Explorer on a computer for this course.
“Exploring Data Sets With R” and “Measuring Relationships and Uncertainty” must be completed prior to starting this course.
- Jan 22, 2025
- Mar 5, 2025
- Apr 16, 2025
- May 28, 2025
- Jul 23, 2025
- Sep 17, 2025
- Nov 12, 2025
How It Works
- View slide #1
- View slide #2
- View slide #3
- View slide #4
- View slide #5
- View slide #6
- View slide #7
- View slide #8
Faculty Authors
Sumanta Basu is an Assistant Professor in the Department of Statistics and Data Science at Cornell University. Broadly, his research interests are structure learning and the prediction of large systems from data, with a particular emphasis on developing learning algorithms for time series data. Professor Basu also collaborates with biological and social scientists on a wide range of problems, including genomics, large-scale metabolomics, and systemic risk monitoring in financial markets. His research is supported by multiple awards from the National Science Foundation and the National Institutes of Health. At Cornell, Professor Basu teaches “Introductory Statistics” for graduate students outside the Statistics Department and “Computational Statistics” for Statistics Ph.D. students. He also serves as a faculty consultant at Cornell Statistical Consulting Unit, which assists the broader Cornell community with various aspects of analyzing empirical research. Professor Basu received his Ph.D. from the University of Michigan and was a postdoctoral scholar at the University of California, Berkeley, and Lawrence Berkeley National Laboratory. Before he received his Ph.D, Professor Basu was a business analyst, working with large retail companies on the design and data analysis of their promotional campaigns.
Kara Karpman is an Adjunct Assistant Professor of Data Science and Statistics at Cornell University, as well as an Assistant Professor of Mathematics at Middlebury College in Middlebury, VT. Her research focuses on statistical modeling techniques for studying biological and financial data. Professor Karpman holds a B.S. in Mathematics from Duke University and an M.S. and Ph.D. in Applied Mathematics from Cornell University.
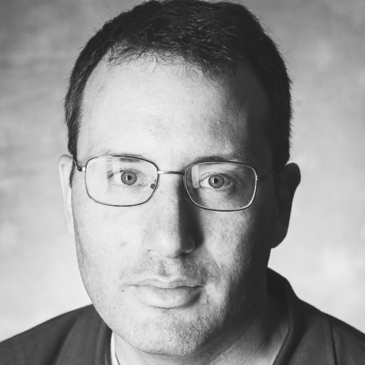
Jeremy Entner, Ph.D., joined Cornell’s Department of Statistics and Data Science as a Lecturer in 2019, where he teaches several courses including “Biological Statistics,” “The Theory of Interest,” and “Statistics for Risk Modeling.” He previously spent six years at the University of Tennessee at Martin teaching courses on mathematics and statistics. Dr. Entner holds a B.S. and M.A. in Mathematics from SUNY Brockport. He earned his Ph.D. in Mathematics with an Emphasis on Statistics from Syracuse University.
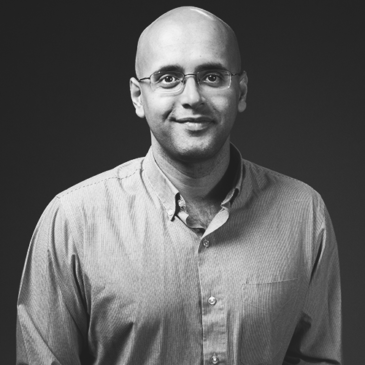
Sumanta Basu is an Assistant Professor in the Department of Statistics and Data Science at Cornell University. Broadly, his research interests are structure learning and the prediction of large systems from data, with a particular emphasis on developing learning algorithms for time series data. Professor Basu also collaborates with biological and social scientists on a wide range of problems, including genomics, large-scale metabolomics, and systemic risk monitoring in financial markets. His research is supported by multiple awards from the National Science Foundation and the National Institutes of Health. At Cornell, Professor Basu teaches “Introductory Statistics” for graduate students outside the Statistics Department and “Computational Statistics” for Statistics Ph.D. students. He also serves as a faculty consultant at Cornell Statistical Consulting Unit, which assists the broader Cornell community with various aspects of analyzing empirical research. Professor Basu received his Ph.D. from the University of Michigan and was a postdoctoral scholar at the University of California, Berkeley, and Lawrence Berkeley National Laboratory. Before he received his Ph.D, Professor Basu was a business analyst, working with large retail companies on the design and data analysis of their promotional campaigns.
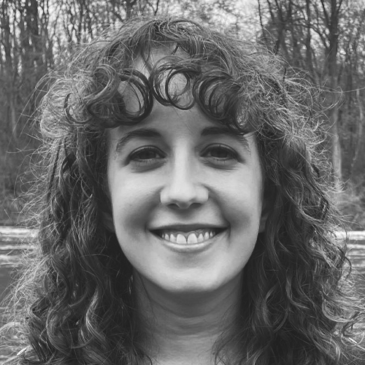
Kara Karpman is an Adjunct Assistant Professor of Data Science and Statistics at Cornell University, as well as an Assistant Professor of Mathematics at Middlebury College in Middlebury, VT. Her research focuses on statistical modeling techniques for studying biological and financial data. Professor Karpman holds a B.S. in Mathematics from Duke University and an M.S. and Ph.D. in Applied Mathematics from Cornell University.
- View slide #1
- View slide #2
- View slide #3
Key Course Takeaways
- Use R to perform mathematical operations, create sets of data, and perform functions on data
- Summarize a data set with the appropriate visualizations and statistics
- Use summarization techniques to interpret data, develop conclusions, and measure the uncertainty of those conclusions
- Answer questions by formulating hypotheses and testing them with real data
- Use simulation techniques to assess uncertainty
- Use linear regression to measure the strength of association between variables
- Clean a data set to answer specific questions
- Create data visualizations for exploratory data analysis and presentations
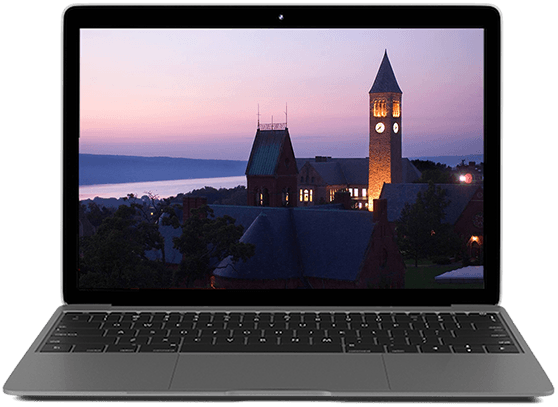
Download a Brochure
Not ready to enroll but want to learn more? Download the certificate brochure to review program details.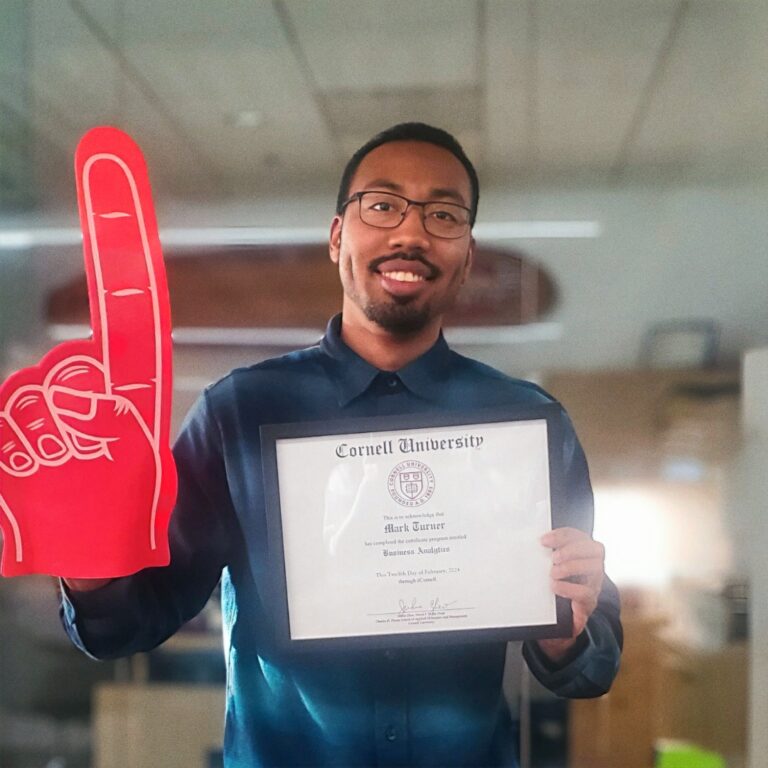
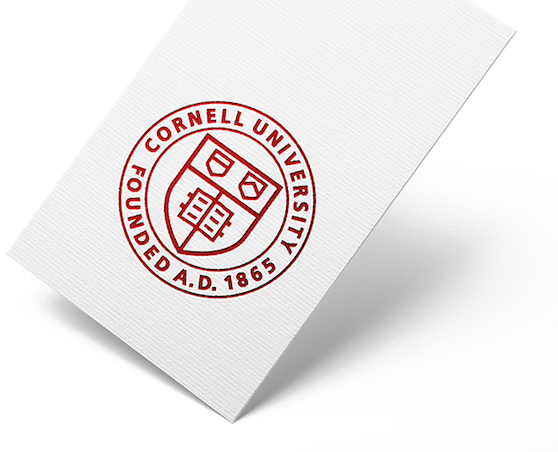
What You'll Earn
- Data Science Essentials Certificate from Cornell Ann S. Bowers College of Computing and Information Science
- 64 Professional Development Hours (6.4 CEUs)
Watch the Video
Who Should Enroll
- Current and aspiring data scientists and analysts
- Business decision makers
- Marketing analysts
- Consultants
- Executives
- Anyone seeking to gain deeper exposure to data science
Explore Related Programs
Request Information Now by completing the form below.
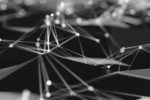
Data Science Essentials
Select Payment Method | Cost |
---|---|
$3,600 | |